Thinking machines
Algorithm-based artificial intelligence, once only imagined, is here. It can do a lot of good.
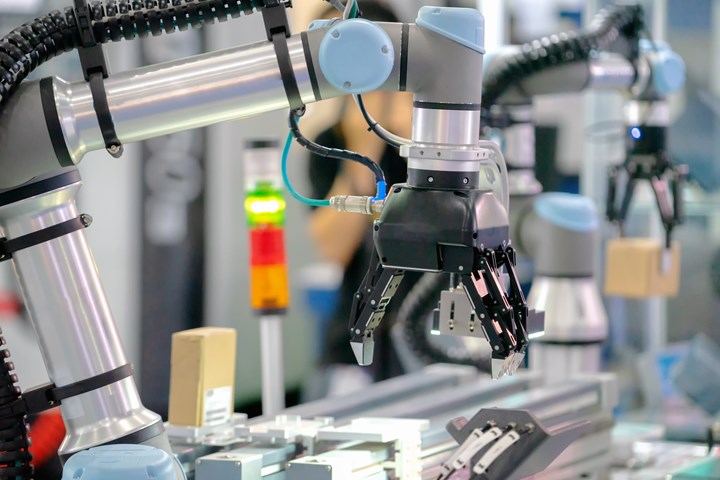
Source | Getty Images
In 1984, when the first Terminator movie came out — featuring Arnold Schwarzenegger as the time-traveling, man/machine assassin — I was a junior in high school and clearly in the movie’s target demographic. Sure enough, I eagerly stood in a long line with several friends at the movie theater the night the movie premiered. And loved it.
If you have not seen Terminator, a quick synopsis: In 2029, computer and machine technology has evolved to outpace and outsmart humans. The machines build weapons to defeat humans and a human-vs.-machines civil war breaks out. The humans are, predictably, losing, but their leader, John Connor, is deemed sufficiently threatening and the machines decide it would be best if John did not exist at all. So, the machines fabricate a human-looking android, a “terminator” (Schwarzenegger), to time-travel back to 1984 to assassinate John’s mother, Sarah, before she can give birth to John. After that, things get complicated in ways you can easily imagine, delivering a variety of sequels along the way.
I remember thinking a couple of things after I saw Terminator. First — setting aside the civil war/time travel premise of the movie — although 2029 seems like a long way away, is it even possible that technology might, over the next 45 years, evolve such that machines could learn and become self-aware and intentional? Second, it seemed to me that learning machines might just as likely be put to use for positive purposes.
Fast-forward to 2020 and, suddenly, 2029 is close enough on the time horizon that we can start to imagine with greater fidelity how technology might evolve. Further, today artificial intelligence (AI), machine learning and evolving algorithms are employed in myriad ways — good and bad, seen and unseen — in manufacturing, healthcare, entertainment, policing, social networking, defense systems and hundreds of other applications. In short, machine learning is here.
What, then, do we make of the threat of machine learning? In 2018, the Pew Research Center published results of a survey (“Artificial Intelligence and the Future of Humans”) of 979 technology thought leaders, basically asking for their assessment of the benefits and threats of algorithm-driven AI. Conveniently and ironically, the survey asked respondents to consider a timeline extending to 2030. AI concerns identified by the survey do not mention a man vs. machine war, but they do include loss of human agency, data abuse, job loss, cognition erosion and mayhem (cybercrime, weaponized drones).
In composites manufacturing, one of the upsides of the growth of algorithm-driven AI systems has been the birth of Industry 4.0 hardware and software, designed to help fabricators manage orders, materials, processes and delivery. It also provides fabricators unprecedented amounts of data about machine operations, process efficiency, product quality, design compliance and much more.
This thread — applied machine learning — runs through three stories in this issue of CW. The first, by senior editor Ginger Gardiner, on p. 30, focuses exclusively on the technologies being developed for what we call Composites 4.0. As Ginger says, “An evolving landscape of automation, sensors and AI software is not an end, but a means to achieve the cost, quality, efficiency and agility required for future manufacturing.”
On p. 16, you will find my report on Spirit AeroSystems’ new RTM production line in Prestwick, Scotland, that will manufacture wing spoilers for the Airbus A320. The focus here is process, but the line is very much enabled by automation and software that governs not just the machines, but the actions of the operators, creating in an audit-compliant digital thread for each spoiler Spirit manufactures.
On p. 28, you will find my story on narrow-tapes ATL technology developed by Fives Lund LLC. While there is much discussion of tape width, a major enabler here is the use of process control technology linked to design allowables for the part, which allows the ATL to strategically and automatically deploy laps and gaps.
All of this is to say that an algorithm-driven, data-driven, machine-smart composites world is not a future notion. It’s a here-and-now and necessary notion. And, properly and thoughtfully employed, it can do a lot of good.
Related Content
PEEK vs. PEKK vs. PAEK and continuous compression molding
Suppliers of thermoplastics and carbon fiber chime in regarding PEEK vs. PEKK, and now PAEK, as well as in-situ consolidation — the supply chain for thermoplastic tape composites continues to evolve.
Read MoreBladder-assisted compression molding derivative produces complex, autoclave-quality automotive parts
HP Composites’ AirPower technology enables high-rate CFRP roof production with 50% energy savings for the Maserati MC20.
Read MoreCompPair adds swift prepreg line to HealTech Standard product family
The HealTech Standard product family from CompPair has been expanded with the addition of CS02, a swift prepreg line.
Read MoreComposite resins price change report
CW’s running summary of resin price change announcements from major material suppliers that serve the composites manufacturing industry.
Read MoreRead Next
VIDEO: High-rate composites production for aerospace
Westlake Epoxy’s process on display at CAMX 2024 reduces cycle time from hours to just 15 minutes.
Read MoreCFRP planing head: 50% less mass, 1.5 times faster rotation
Novel, modular design minimizes weight for high-precision cutting tools with faster production speeds.
Read MoreModeling and characterization of crushable composite structures
How the predictive tool “CZone” is applied to simulate the axial crushing response of composites, providing valuable insights into their use for motorsport applications.
Read More