Digital thread vs. digital twin
Visualizing the difference between digital representations and data flows and understanding the applications that are changing composites manufacturing and business models.

Source | CW, nebumind, Dynexa
This is an online sidebar for the July 2020 article, “Composites 4.0: Digital transformation, adaptive production, new paradigms”.
When I first discussed these concepts in 2016, they were still somewhat nascent in composites. Industry 4.0 has evolved since then, with more composites manufacturers and production lines using them and more software creating and managing them (see “Composites 4.0 architecture and ontology”). However, to me, digital twin and digital thread are often referenced in a way that makes them seem interchangeable. They are, instead, very distinct.
Digital model vs. communication framework
Digital twin: a virtual model of each physical production asset — i.e., each jet engine or wind turbine (see 2016 blog, “Digital twin, digital thread and composites”). Adding to that and contrasting with the digital thread, I draw from Conrad Leiva’s 2016 IndustryWeek.com article “Demystifying the Digital Thread and Digital Twin Concepts”:
The digital twin refers to a digital model of a particular asset that includes design specifications and engineering models describing its geometry, materials, components and behavior. More important, it also includes the as-built and operational data unique to the specific physical asset that it represents. For example, for an aircraft, the digital twin would be identified to the physical product unit identifier which is referred to as the tail number.
… The digital thread refers to the communication framework that allows a connected data flow and integrated view of the asset’s data throughout its lifecycle across traditionally siloed functional perspectives. The digital thread concept raises the bar for delivering “the right information to the right place at the right time.”
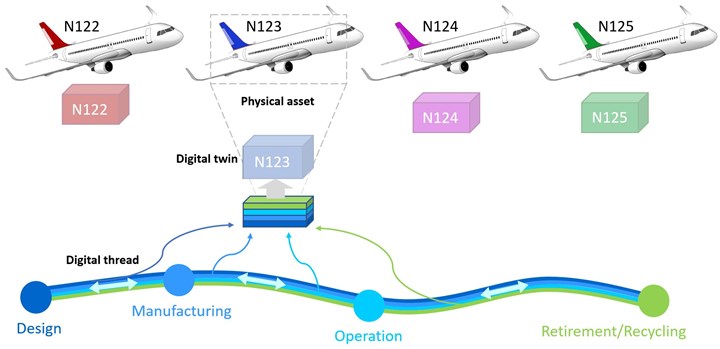
The digital twin is the design, as-built manufacturing and operational data for each physical asset — aircraft with tail number N123 has its specific and unique digital twin N123. The digital thread enables bidirectional flow of data — forward to build the digital twin and also feedback to continuously improve design, manufacturing, operation, etc. Source | CW
Another illustration is the digital thread for additive manufacturing (DTAM, below), as envisioned by consulting firm Deloitt. The digital thread begins with product design, which is then incorporated into the digital twin. Both proceed through analysis, simulation of part and process, part fabrication, post-processing, inspection and field service, with the digital twin growing larger as data is added at each stage, while the digital thread enables communication of that data forward and backward through the process and supply chain.
Deloitte doesn’t show the digital thread extending to end-of-life recycling. But for composite parts, this would be vital to provide the pedigree for recycled fibers and compounds which would then help comprise the digital twin and thread for future parts made with those materials.
Digital thread for additive manufacturing (DTAM)
Source | Deloitte analysis, deloitte.com/insights
Digital twin as digital database
In the Deloitte illustration above, the digital twin is described as a “body of knowledge” that grows throughout the life of a part. Thus, the digital twin is often referred to as a digital database. The ZAero project provides an example of how this applies to composites. Aimed to improve the efficiency of making composite aerostructures like wingskins, ZAero’s partners worked to integrate sensors into the automated layup, resin infusion and curing processes used to make carbon fiber-reinforced polymer (CFRP) stiffened panel demonstrators (see main article and “ZAero project update”).
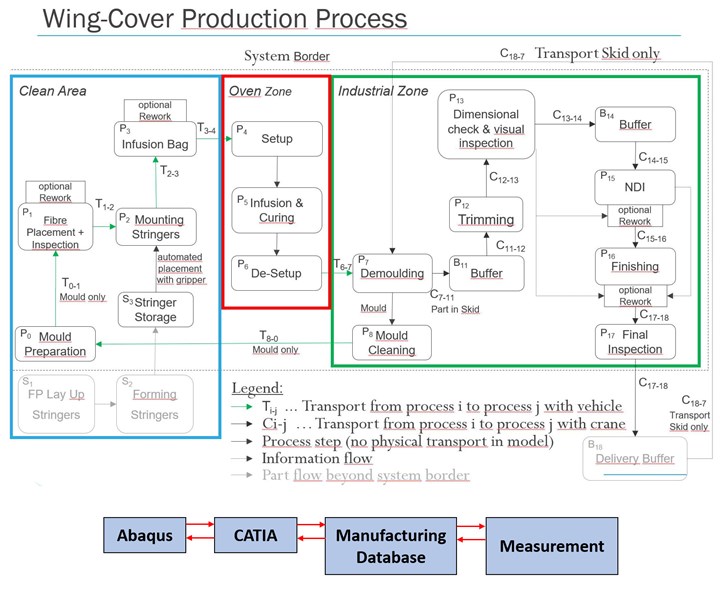
Data flow during CFRP wingskin production process (top) and steps in the ZAero project’s inline automatic control system (bottom) to detect defects and guide rework during CFRP stiffened panel manufacture. Source | ZAero, Profactor
The defects detected by these sensors were then input into part performance simulations to guide rework decisions. In addition to sensors that can detect standard defects during automated fiber placement (AFP), says Dr. Christian Eitzinger, ZAero project manager at Profactor (Steyr, Austria), “We now have three different sensors that measure three different process parameters — temperature, state of cure and resin flow front — during infusion. We have tested and integrated these with CATIA 3D Experience software and shown that the data can be reliably acquired and added to the part’s database. Ideally, this digital database begins during the part design and extends through material acquisition and preparation, layup, curing and then final NDT [nondestructive testing], so that it is a full and accurate representation of the part.”
In the real-life application envisioned by the ZAero project, that full and accurate representation would be for an actual wingskin and would include any rework details, including locations within the part that were repaired, materials and processes used, process parameters, NDT results, etc. All of this too would become part of that wingskin’s digital database/digital twin.
Process and inspection data was also collected for each part in the iComposite 4.0 project (see main article and 2018 blog, plus slideshow below), which was completed at AZL Aachen Center for Integrative Lightweight Production (AZL) at RWTH Aachen University (Aachen, Germany). For this project, which sought to reduce the cost of automotive parts, it was envisioned that each part’s production data would be stored in radio frequency identification (RFID) chips embedded in the part. Thus, the RFID chip provides a means for the digital thread, communicating that part’s data as it is scanned prior to final assembly, for example. “We have found that a QR code or similar works just as well,” says Dr. Michael Emonts, managing director at AZL. “It is linked to a database where all of the part’s process and measurement data is stored.”
Note that other sensors are available, such as the Bluetooth tags being used by aerospace tier Kanfit (Nof-Hagalil, Israel) to track location and progress of parts on the factory floor. The tags are part of a system that uses artificial intelligence (AI) to alert when a part is straying from its work order but also to suggest part flow improvements. It has even influenced how Kanfit designed its newest facility (see “Kanfit … managing growth using Composites 4.0 systems”).
iComposite 4.0 project
Applications and considerations
Dynexa (Laudenbach, Germany) produces composite tubes and shafts using a wet winding process. Having completed its first steps toward digital transformation (see main article), the company is now working to provide a digital ecosystem for its customers. In addition to creating an online portal where customers use Dynexa’s automated software to specify, customize, price and order their parts in minutes vs. days, the company is also responding to requests for replacing paper data sheets with digital labels.
“We have all of the part’s design and production data,” explains Dynexa managing director Christian Koppenberg, “so, now we are collecting that into a label on the part which the customer can scan.” From GE forward, digital twin has long been touted as a key to predictive maintenance. “Actually, our customers said they don’t need predictive maintenance,” notes Koppenberg, “but instead all of the part data in one place, including quality control sheets and handling guidelines.”
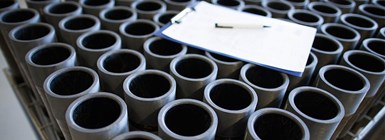
Dynexa collects each part’s data into a label on the part which the customer can scan. Source | Dynexa
He concedes the company had to work through whether this would be a QR code, bar code or RFID chip, and also where to place these on the part to survive the curing process. But Dynexa is a good example of how even the beginnings of using digital twin can be used to meet customer needs and increase efficiency and competitiveness along the supply chain.
Another interesting application is use of this digital database/digital twin to prove quality of a component prior to shipping and installation into a larger automotive or aircraft assembly. During various discussions about this topic, I was told about one Tier supplier whose customer repeatedly had issues with their parts during assembly. The Tier reiterated the quality of the part as-shipped and suspected the issues were caused with damage during subsequent handling. Its solution was to embed sensors into its parts which collect data after leaving the factory. By combining Dynexa’s approach with these post-factory sensors, it is possible to now have a digital twin that not only documents and verifies the component’s as-produced quality and characteristics, but also, for example, temperature and forces applied during subsequent shipping, handling and installation.
The composites industry has long discussed structural health monitoring (SHM), which has mostly focused on predictive maintenance. But the example above gives a more near-term application that directly drives profitability along the supply chain. It not only protects the composites part manufacturer, it also helps the next tier/OEM to identify possible issues in its procedures and/or workforce training.
Digital thread = connected supply chain
The examples above illustrate how the digital thread both enables digital twins and connects the supply chain with significant impact. In my 2016 blog on digital twin and digital thread, I explained how General Electric (GE, Boston, Mass., U.S.) had begun using sensors and big data analytics to improve both the speed and efficiency of manufacturing:
“It sees a self-improving, agile and connected supply chain communicating and operating through a digital thread in real time.”
“This is indeed what we are helping to establish,” says Avner Ben-Bassat, president of Plataine (Waltham, Mass., U.S. and Tel Aviv, Israel), a supplier of Industrial Internet of Things (IoT) software for composites manufacturing. “We are helping our customers to collect data on all parts, kits, processes, machines and tools in real time and then store that data, enabling digital twins and complete traceability. Parts can be shipped by the Tier 2 supplier to the Tier 1, where further data is added, and then on to the OEM. This is where we are headed: a connected, digital supply chain. Moreover, the stored data is accessible anytime for quick damage control and audits.”
Plataine software accepts data from Virtek layup projectors and TE Wire & Cable thermocouples to create digital twins and context-aware alerts and recommendations. Source | Plataine, Virtek, TE Wire & Cable
What kind of data and sensors can Plataine’s software accommodate? Thecompany began with prepreg cut planning and tracking prepreg out-time. It now includes tool tracking and AI-based digital assistants throughout production. “Plataine looks at a whole spectrum of sensors and what our customers want to accomplish,” says Ben-Bassat. “For example, we have worked with Virtek (Waterloo, ON, Canada) to integrate our software into their layup projectors. This then extends reliable, real-time traceability to layup. We also know what the status is during a specific layup job which, for example, may impact scheduling for the next autoclave run. We can also check if that station is laying up on the right tool. However, the prize is the ability to not only track production but to influence it.”
Ben-Bassat continues, “Integrating projectors gives us a very accurate handle on layup in real time, but now you can also perform analysis. We see not just an alert that the layup is taking longer than it should, but we can ask: ‘Will the prepreg expire soon? Is it the operator going too slow? Is it an issue with the tool or the part design?’ We are identifying problems before they affect quality and enabling informed solutions, which in turn drives continuous improvement.” He notes that this requires the support of AI software and enables a fast response to potential problems, even before they occur. Just as demonstrated in the iComposite 4.0 and ZAero projects, this is reaction much earlier in the process chain versus waiting until after cure and final NDT. The result is significant improvement in part quality and quality control, rework, cost and on-time performance.
Plataine can also integrate data from AFP laser sensors that detect defects and laser projectors that show where they are located. “We can now add this to each part’s digital twin and to the digital thread for a production line or complete factory,” says Ben-Bassat. However, he cautions, “to gain the full value of this data, it must be constantly analyzed in real-time to deliver alerts, insights and recommendations.”
All of this data is stored in the cloud. But as I discussed in my 2019 blog on digital assistants and edge computing, Plataine also has software that enables crunching data at the edge of the devices where it is gathered instead of long routing to cloud servers and back. This cuts the distance between data generation, processing and feedback into the IoT system, which then minimizes latency, an imperative for Composites 4.0 production lines to operate in real time.
“It’s not that cloud computing power is eliminated, it’s more that it is being distributed to the production line.” — Plataine
Digital twin for product vs. production
And this brings us to one final point: a digital twin can be developed not just for a product but also for its production — in other words, for each production line or factory. To differentiate between product and production digital twins, I will explore new software by nebumind (Munich, Germany).
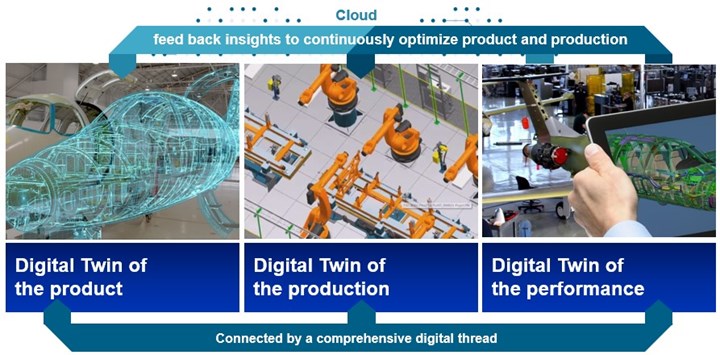
Source | Siemens PLM
Like Plataine, nebumind supplies IoT software for manufacturing that enables a digital twin of the part produced by machines. “Our specialty, however, is to map production data onto every location of the part,” explains Franz Engel, nebumind co-CEO and co-founder with Caroline Legler. Both CEOs previously managed the Airbus subsidiary InFactory Solutions, developing sensors for AFP inline inspection and resin infusion. “Many digital twins are of the production,” Engel continues, “analyzing manufacturing flow and efficiency. Ours is a more part-centric view.”
nebumind’s software builds a 3D model of each part produced and adds all machine and sensor data generated during its manufacture. For now, the software works with CNC and robot-based processes such as AFP, 3D printing and milling/drilling. “For every machine position on the part, we collect the process data such as temperature, speed and pressure,” explains Engel. “For all data that is recorded, we collect and store also the time when it was recorded and, more importantly, the location or position on the part where it was recorded. Now, when we analyze part quality and see a defect at point x, y, z, but only on every fifth part, we can look in our software at all of the parts produced and see all of the parameters for just that location. We can see how the parameters compare between parts with and without defects, and also how that location compares to the rest of the part. In this way, we can trace back quality defects to their origin and control part quality across manufacturing process steps.”
“nebumind is geared toward providing a high level of granularity for a single process,” says Florian Krebs, team leader for flexible automation at the German Aerospace Center (DLR) Center for Lightweight Production Technology (ZLP, Augsburg, Germany). Krebs is part of the team that has developed an AI-equipped work cell where collaborative robots can switch from producing composite rear pressure bulkheads to fuselage panels without requiring reprogramming or retraining (see main article and “No business case for reteaching robots”). “nebumind provides location-based data collection and storage, as well as automated analytics to predict part quality and gain confidence in causality of that quality. It also enables a visual representation of the data. We are looking at integrating nebumind into our central storage to help with our reports.”
That central storage is the digital twin.“The most important point of digital twin is to have one central repository, one source of truth,” says Dr. Michael Kupke, head of the ZLP Augsburg. The diagram below shows the data architecture for the ZLP’s flexible automation platform that allows the AI-equipped work cell to build a CFRP rear pressure bulkhead (upper right corner) or fuselage panel (lower right) and switch between quickly. Note the central storage/repository is the gray disk at center marked Data Base. “We collect all of the data into this central repository to improve process quality but also for visualization,” says Krebs.
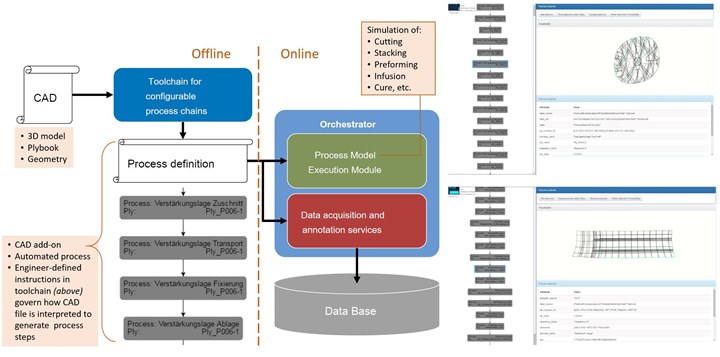
For the PROTEC NSR and Factory of the Future project, ZLP developed a flexible automation platform that can produce a CFRP rear pressure bulkhead (top right) or fuselage panel (bottom right), and switch between these quickly by simply changing the CAD file. For enlarged image, see main article. Source | DLR Institute for Structures and Design
“There are many software solutions to collect data and put it in a digital twin,” says Krebs, “for example from Google, Amazon and Microsoft. The key is to tailor these to your requirements.” Engel contends these solutions don’t go as deep into the process of making the composite part as nebumind does. “We help to understand defects and find the root cause. We make it easy and fast to identify the source of the defect in the manufacturing data and also to monitor this online. For example, you can set an alarm if temperature in the upper right corner exceeds 100°C.”
“Plataine is trying to improve the entire part-making process,” says Ben-Bassat. “nebumind could provide a very useful data source that we could roll in to make the digital thread richer and more informed.”
Stay tuned for my full blog on nebumind, and, I’m sure, future updates on Plataine, ZLP, AZL and other providers of Composites 4.0 tools and solutions.
Related Content
Composites end markets: Electronics (2024)
Increasingly, prototype and production-ready smart devices featuring thermoplastic composite cases and other components provide lightweight, optimized sustainable alternatives to metal.
Read MoreMicrowave heating for more sustainable carbon fiber
Skeptics say it won’t work — Osaka-based Microwave Chemical Co. says it already has — and continues to advance its simulation-based technology to slash energy use and emissions in manufacturing.
Read MoreHexagon Purus Westminster: Experience, growth, new developments in hydrogen storage
Hexagon Purus scales production of Type 4 composite tanks, discusses growth, recyclability, sensors and carbon fiber supply and sustainability.
Read MoreCirculinQ: Glass fiber, recycled plastic turn paving into climate solutions
Durable, modular paving system from recycled composite filters, collects, infiltrates stormwater to reduce flooding and recharge local aquifers.
Read MoreRead Next
Plant tour: Daher Shap’in TechCenter and composites production plant, Saint-Aignan-de-Grandlieu, France
Co-located R&D and production advance OOA thermosets, thermoplastics, welding, recycling and digital technologies for faster processing and certification of lighter, more sustainable composites.
Read MoreVIDEO: High-volume processing for fiberglass components
Cannon Ergos, a company specializing in high-ton presses and equipment for composites fabrication and plastics processing, displayed automotive and industrial components at CAMX 2024.
Read More“Structured air” TPS safeguards composite structures
Powered by an 85% air/15% pure polyimide aerogel, Blueshift’s novel material system protects structures during transient thermal events from -200°C to beyond 2400°C for rockets, battery boxes and more.
Read More