Using machine learning to accelerate composites processing simulation
A speed gain of 1,000 to 10,000 times greater than traditional FE models has been achieved using machine learning models, enabling near real-time simulation for large composite components.
Share
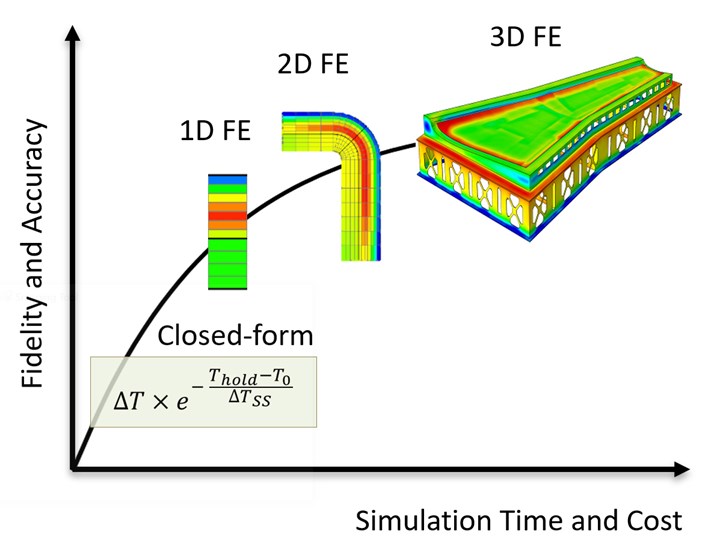
Fig. 1. Trade-off between fidelity/accuracy and simulation time for different solutions providing thermo-chemical analysis of composite parts during processing. Photo Credit: Convergent Manufacturing Technologies.
Processing fiber-reinforced polymer (FRP) composites is a complex and multiphysics problem of heat and mass transfer, thermo-chemical phase transitions and highly nonlinear and time-dependent viscoelastic stress developments. To reduce manufacturing risks and overall production cost, more emphasis has recently been placed on process simulation to mitigate defects rather than relying on know-how and trial-and-error. This digitally empowered approach typically uses general-purpose commercial finite element (FE) simulation tools such as ABAQUS (Dassault Systèmes, Vélizy-Villacoublay, France) or ANSYS (Canonsburg, Pa., U.S.), along with specialized FE tools such as COMPRO (Convergent Manufacturing Technologies, Vancouver, B.C., Canada) that include built-in material libraries to represent the complex evolution of composites properties during processing.
Current industrial implementation of this approach relies on conducting costly 3D FE analysis of the part and tool, which are subjected to convective heating. During the preliminary design phase, many design iterations are needed to finalize details such as part thickness, layup, cure cycle and tooling material and geometry. For a given large component, such as a composite wing skin, an analysis using 3D FE simulation may take weeks
To accelerate process simulation, reduced-order FE can be used instead of 3D FE at the preliminary design phase. For thermo-chemical analysis of composites, for example, the dominant heat transfer mechanism for thin parts is through-the-thickness, away from the edges and tool substructures. Thus, 1D FE analysis can be conducted as a good approximation of the part’s 3D response, and a complex part may be divided into unique zones to perform several 1D FE analyses rather than a full 3D FE across the part. This speeds up process simulation at the preliminary design phase. There is, however, the speed-fidelity trade-off as demonstrated in Fig. 1.
Moving beyond the preliminary design toward the detailed design, however, requires completion of a number of 3D FE simulations. Even using software that enables reduced-order process simulation, such as the Composites Producibility Assessment – Thermal Analysis (CPA-TA) by Convergent Manufacturing Technologies, simulation of a large composite component may still require several minutes to an hour. Although this is a significant gain compared to full 3D FE, it is still not fast enough to efficiently explore the entire design spectrum for optimization.
Replacing FE with machine learning
Given the recent advances in machine learning (ML) and data-driven methods, many branches of science and engineering have started implementing ML for different applications. One emerging application is to train fast surrogate ML models to replace slow FE simulation tools. In this approach, FE models are used to automatically generate a large amount of data based on different assumptions for input parameters. This data then can be used to train different ML models such as neural networks (NN), random forest models or Gaussian Process Regression (GPR) models. A properly trained surrogate model can closely replicate the FE model used to train it, but offers a significant gain in simulation speed.
In a recent collaborative effort between Professor Navid Zobeiry from the University of Washington, Professor Anoush Poursartip from the University of British Columbia and a team from Convergent Manufacturing Technologies, surrogate ML models were developed in addition to reduced-order FE modeling to speed up composites processing simulations. In this study, surrogate NN models were developed to replace reduced-order FE. These models were trained using data generated from FE simulations using Convergent Manufacturing Technologies’ RAVEN software, as well as the theory governing the curing problem of composites (i.e., theory-guided machine learning or TGML). These NN models can use the same inputs as FE simulations to predict key performance indicators during composites processing, including the maximum part temperature during the exothermic curing reaction. On average, using a typical computer workstation, a speed gain of 1,000 to 10,000 times greater than FE models was achieved using these ML models. This has enabled near real-time simulation speed for large composite components.
Fig. 2. Comparison of simulation times using different simulation approaches for thermo-chemical analysis of a representative composite part on an Invar tool. Photo Credit: Convergent Manufacturing Technologies
These surrogate NN models were recently implemented in CPA-TA by Convergent Manufacturing Technologies and their performance was demonstrated for process simulation of a large composite wing skin. A representative 5 x 10-meter wing skin design is shown in Fig. 2 with a representative ply definition comprising 40 to 80 plies in thickness, as well as pad-ups, ply drops and other features in a typical wing skin. Process simulation of the wing skin on a representative production tool took several hours using 3D FE on a typical computer workstation using the commercial software package COMPRO in ABAQUS. It is noteworthy that process optimization using this approach will take days or weeks. On a second attempt, the wing skin was divided into 30 unique 1D geometries. Using reduced-order FE, nine simulations were conducted for the entire wing skin using three temperature cycles and three tool thicknesses for optimization. These simulations using CPA-TA in CATIA 5 took about half an hour on the same computer which was used for 3D FE.
In a final demonstration, the same simulation was conducted using trained NN models in CPA-TA with extraordinary results. Similar to reduced-order FE, the wing skin was divided into 30 zones and nine simulations were conducted with different tooling thicknesses and air temperature profiles. However, these simulations were completed in only two seconds. A comparison of these simulation approaches (Fig. 2) clearly demonstrates the capability of ML methods to achieve near real-time simulation capability and drastic time savings for complex composite structures. Notably, the success of the techniques discussed here can be applied to other engineering applications as well.
About the Author
Navid Zobeiry
Navid Zobeiry is an assistant professor of Materials Science & Engineering at the University of Washington (Seattle, Wa., U.S.). He was previously a research associate at the University of British Columbia, where he led manufacturing and testing for the Composites Research Network. Throughout his research career, he has collaborated extensively with aerospace and automotive manufacturers as well as materials suppliers on a wide range of topics including material and process characterizations, process simulation and optimization, manufacturing-induced defects and theory-guided machine learning.
Related Content
Plant tour: Joby Aviation, Marina, Calif., U.S.
As the advanced air mobility market begins to take shape, market leader Joby Aviation works to industrialize composites manufacturing for its first-generation, composites-intensive, all-electric air taxi.
Read MoreNext-generation airship design enabled by modern composites
LTA Research’s proof-of-concept Pathfinder 1 modernizes a fully rigid airship design with a largely carbon fiber composite frame. R&D has already begun on higher volume, more automated manufacturing for the future.
Read MorePEEK vs. PEKK vs. PAEK and continuous compression molding
Suppliers of thermoplastics and carbon fiber chime in regarding PEEK vs. PEKK, and now PAEK, as well as in-situ consolidation — the supply chain for thermoplastic tape composites continues to evolve.
Read MoreOne-piece, one-shot, 17-meter wing spar for high-rate aircraft manufacture
GKN Aerospace has spent the last five years developing materials strategies and resin transfer molding (RTM) for an aircraft trailing edge wing spar for the Airbus Wing of Tomorrow program.
Read MoreRead Next
The next-generation single-aisle: Implications for the composites industry
While the world continues to wait for new single-aisle program announcements from Airbus and Boeing, it’s clear composites will play a role in their fabrication. But in what ways, and what capacity?
Read MorePlant tour: Teijin Carbon America Inc., Greenwood, S.C., U.S.
In 2018, Teijin broke ground on a facility that is reportedly the largest capacity carbon fiber line currently in existence. The line has been fully functional for nearly two years and has plenty of room for expansion.
Read More“Structured air” TPS safeguards composite structures
Powered by an 85% air/15% pure polyimide aerogel, Blueshift’s novel material system protects structures during transient thermal events from -200°C to beyond 2400°C for rockets, battery boxes and more.
Read More